Programme
SHARP will be held on 19 June 2022. The workshop will follow a hybrid format.
The program will be as follows (CDT time zone):
Opening – Prof. Djamila Aouada |
13:30 – 13:35 |
Presentation of SHARP Challenges – Prof. Djamila Aouada |
13:35 – 13:50 |
Plenary Talk – Prof. Angela Dai (onsite live talk) |
13:50 – 14:40 |
Coffee Break |
14:40 – 14:55 |
Finalists 1: Points2ISTF – Implicit Shape and Texture Field from Partial Point Clouds – Jianchuan Chen |
14:55 – 15:15 |
Finalists 2: 3D Textured Shape Recovery with Learned Geometric Priors – Lei Li |
15:15 – 15:35 |
[Challenge 2 organizer’s baseline] – Parametric Sharp Edges from 3D Scans – Elona Dupont |
15:35 – 15:55 |
Plenary Talk – Prof. Tolga Birdal (onsite live talk) |
15:55 – 16:45 |
Announcement of Results – Artec3D |
16:45 – 16:55 |
Analysis of Results – Dr. Anis Kacem |
16:55 – 17:20 |
Demo by Artec3D + Closing Remarks (Prof. Djamila Aouada) |
17:20 – 17:35 |
Invited Speakers
Plenary Talks
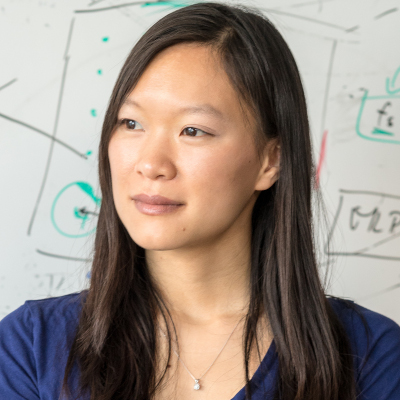
Prof. Angela Dai
Technical University of Munich
Bio: Angela Dai is an Assistant Professor at the Technical University of Munich where she leads the 3D AI group. Prof. Dai’s research focuses on understanding how the 3D world around us can be modeled and semantically understood. Previously, she received her PhD in computer science from Stanford in 2018 and her BSE in computer science from Princeton in 2013. Her research has been recognized through a Eurographics Young Researcher Award, ZDB Junior Research Group Award, an ACM SIGGRAPH Outstanding Doctoral Dissertation Honorable Mention, as well as a Stanford Graduate Fellowship.
Title: Towards Commodity 3D Content Creation.
Abstract: With the increasing availability of high quality imaging and even depth imaging now available as commodity sensors, comes the potential to democratize 3D content creation. State-of-the-art reconstruction results from commodity RGB and RGB-D sensors have achieved impressive tracking, but reconstructions remain far from usable in practical applications such as mixed reality or content creation, since they do not match the high quality of artist-modeled 3D graphics content: models remain incomplete, unsegmented, and with low-quality texturing. In this talk, we will address these challenges: I will present a self-supervised approach to learn effective geometric priors from limited real-world 3D data, then discuss object-level understanding from a single image, followed by realistic 3D texturing from real-world image observations. This will help to enable a closer step towards commodity 3D content creation.
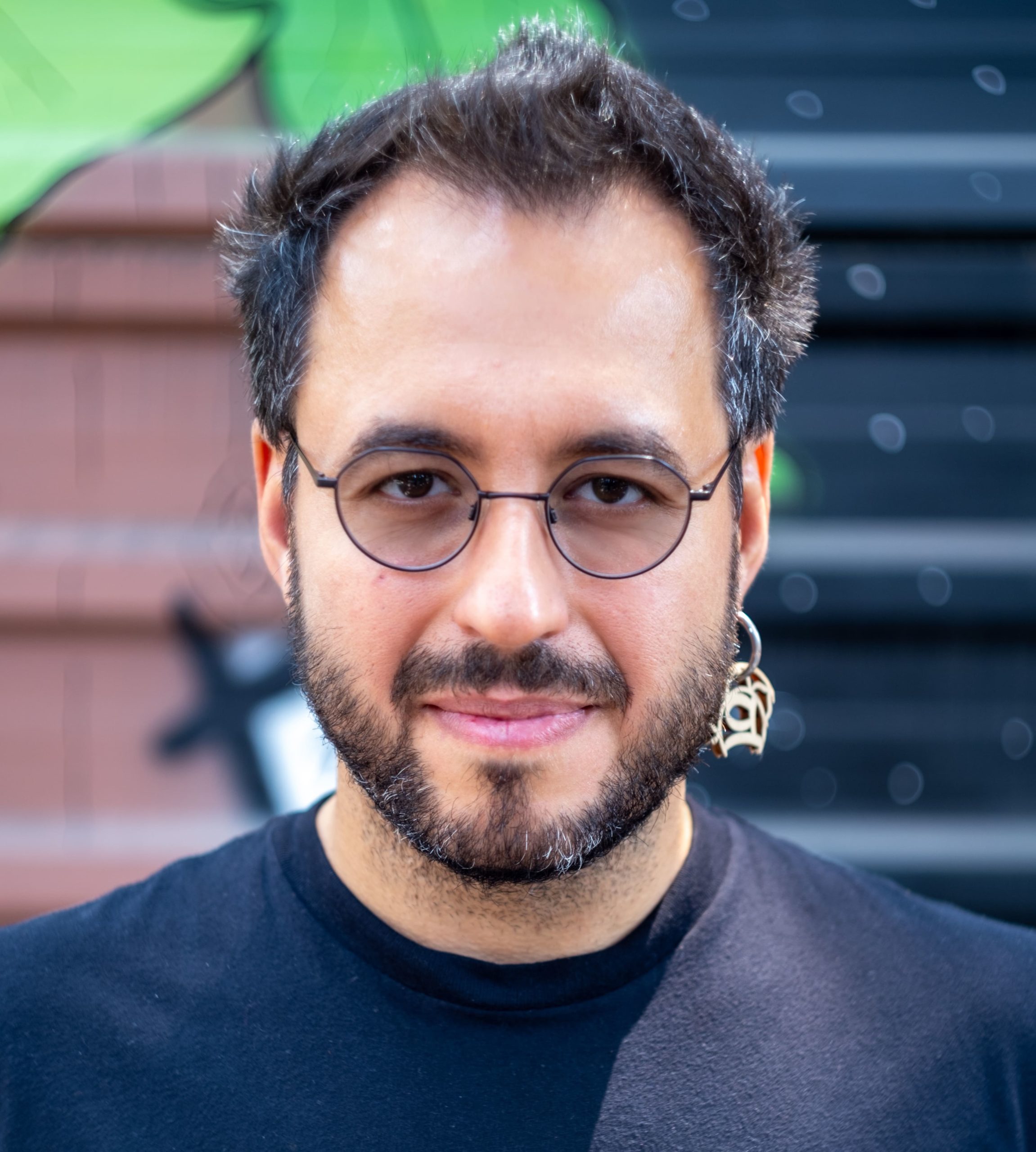
Prof. Tolga Birdal
Imperial College London
Bio: Tolga Birdal is an assistant professor in the Department of Computing of Imperial College London. Previously, he was a senior Postdoctoral Research Fellow at Stanford University within the Geometric Computing Group of Prof. Leonidas Guibas. Tolga has defended his masters and Ph.D. theses at the Computer Vision Group under Chair for Computer Aided Medical Procedures, Technical University of Munich led by Prof. Nassir Navab. He was also a Doktorand at Siemens AG under supervision of Dr. Slobodan Ilic working on “Geometric Methods for 3D Reconstruction from Large Point Clouds”. His current foci of interest involve geometric machine learning and 3D computer vision. More theoretical work is aimed at investigating and interrogating limits in geometric computing and non-Euclidean inference as well as principles of deep learning. Tolga has several publications at the well-respected venues such as NeurIPS, CVPR, ICCV, ECCV, T-PAMI, ICRA, IROS, ICASSP and 3DV. Aside from his academic life, Tolga has co-founded multiple companies including Befunky, a widely used web-based image editing platform.
Title: Rigid & Non-Rigid Multi-Way Point Cloud Matching via Late Fusion
Abstract: Correspondences fuel a variety of applications from texture-transfer to structure from motion. However, simultaneous registration or alignment of multiple, rigid, articulated or non-rigid partial point clouds is a notoriously difficult challenge in 3D computer vision. With the advances in 3D sensing, solving this problem becomes even more crucial than ever as the observations for visual perception hardly ever come as a single image or scan. In this talk, I will present an unfinished quest in pursuit of generalizable, robust, scalable and flexible methods, designed to solve this problem. The talk is composed of two sections diving into (i) MultiBodySync, specialized in multi-body & articulated generalizable 3D motion segmentation as well as estimation, and (ii) SyNoRim, aiming at jointly matching multiple non-rigid shapes by relating learned functions defined on the point clouds. Both of these methods utilize a family of recently matured graph optimization techniques called synchronization as differentiable modules to ensure multi-scan / multi-view consistency in the late stages of deep architectures. Our methods can work on a diverse set of datasets and are general in the sense that they can solve a larger class of problems than the existing methods.