SPARK Challenge : SPAcecraft Recognition leveraging Knowledge of Space Environment
NEW!: Challenge at the IEEE International Conference on Image Processing (ICIP)
Submissions & Evaluation Webinar Video (04.06.2021)
Brief Introduction
Acquiring information and knowledge about objects orbiting around earth is known as Space Situational Awareness (SSA). SSA has become an important research topic thanks to multiple large initiatives, e.g., from the European Space Agency (ESA), and from the American National Aeronautics and Space Administration (NASA).
Vision-based sensors are a great source of information for SSA, especially useful for spacecraft navigation and rendezvous operations where two satellites are to meet at the same orbit and perform close-proximity operations, e.g., docking, in-space refuelling, and satellite servicing. Moreover, vision-based target recognition is an important component of SSA and a crucial step towards reaching autonomy in space. However, although major advances have been made in image-based object recognition in general, very little has been tested or designed for the space environment.
State-of-art object recognition algorithms are deep learning approaches requiring large datasets for training. The lack of sufficient labelled space data has limited the efforts of the research community in developing data-driven space object recognition approaches. Indeed, in contrast to terrestrial applications, the quality of spaceborne imaging is highly dependent on many specific factors such as varying illumination conditions, low signal-to-noise ratio, and high contrast.
We propose a challenge to design data-driven approaches for space target recognition, including classification and detection. The proposed SPARK challenge launches a new unique space multi-modal annotated image dataset. The SPARK dataset contains a total of ∼150k RGB images and the same number, ∼150k, of depth images of 11 object classes, with 10 spacecrafts and one class of space debris.
The data have been generated under a realistic space simulation environment, with a large diversity in sensing conditions, including extreme and challenging ones for different orbital scenarios, background noise, low signal-to-noise ratio (SNR), and high image contrast that defines actual space imagery.
This challenge offers an opportunity to benchmark existing object classification and recognition algorithms, including multi-modal approaches using both RGB and depth data. It intends to intensify research efforts on automatic space object recognition and aims to spark collaborations between the image/vision sensing community and the space community.
Rules of participation
The challenge considers 11 classes (10 spacecrafts and 1 class for debris). Each image contains one class only. Depth images taken simultaneously are also provided and can be used as additional information. They are provided to offer the possibility to investigate the impact of multi-modal recognition.
The SPARK challenge proposes two (tightly) related tasks that will be evaluated under three different sensing conditions:
Task 1 – Classification
Task 2 – Detection
How to participate
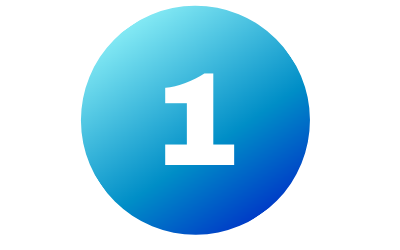
Participants should register and send back a signed Data License Agreement before the deadline of April 15th, 2021. They will be asked to provide their name, contact information and affiliation. The registration is nonbinding.
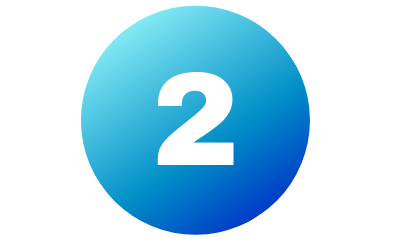
A training dataset composed of 90k RGB images and 90k depth images with class labels and bounding boxes will be provided on April 15, 2021. Participants are allowed to use data augmentation techniques and any other datasets for training as long as this information is reported.
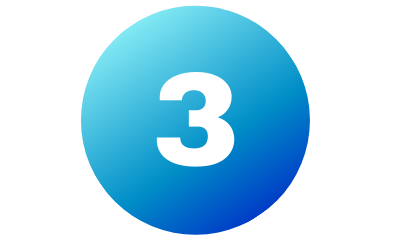
Access to the validation dataset (with ground truth labels and bounding boxes) will be granted on April 30, 2021.
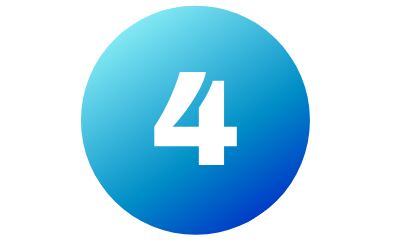
One testing set (with no ground truth labels, nor bounding boxes) will be provided to all participants on June 7, 2021. This dataset represents 3 different levels of complexity with increasing challenges emulating real space sensing conditions.
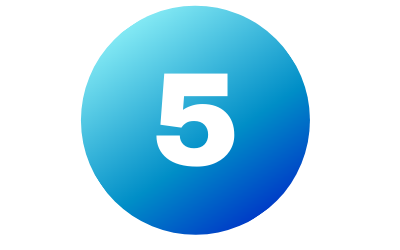
Results for each testing set should be submitted using a predefined format. An example will be provided on the challenge Gitlab page. Each participant will be allowed to submit up to 5 results before the deadline of June 10, 2021. Only the best result will be counted towards the competition.
Criteria of judging a submission
The submissions for the two tasks will be evaluated as follows:
- Classification: In this case, a specific problem-oriented metric will be computed.
- Detection: In this case, in addition to classification, localisation is also evaluated. The standard evaluation procedure used for the COCO challenge will be used.
Dataset
The SPARK dataset is a unique and new space dataset generated using the Unity3D game engine as a simulation environment. A detailed description of the SPARK data and its statistical analysis will be published.
A webpage allowing to request access to the dataset will be available on the following dedicated website https://cvi2.uni.lu/datasets/. Upon signing a Data License Agreement, requestors will be given access to the data.
The simulation environment models:
This model represents the observer that is equipped with different vision systems.
A pinhole camera model was used with known intrinsic camera parameters and optical sensor specifications.
The SPARK dataset was generated by randomly placing the target satellite or spacecraft within the field of view of the camera mounted on the chaser. Different ranges and orientations of the chaser model were simulated. Furthermore, sun and earth were randomly rotated around their axes in every frame. The final SPARK dataset consists of ∼150k high-resolution photo-realistic RGB images, ∼150k depth images and the corresponding ∼150k bounding boxes in multiple and different space environmental conditions, with 10 different satellites and 5 other debris objects.
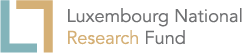