25th June 2021 - 4:00 p.m. - 8:30 p.m. CET
The program
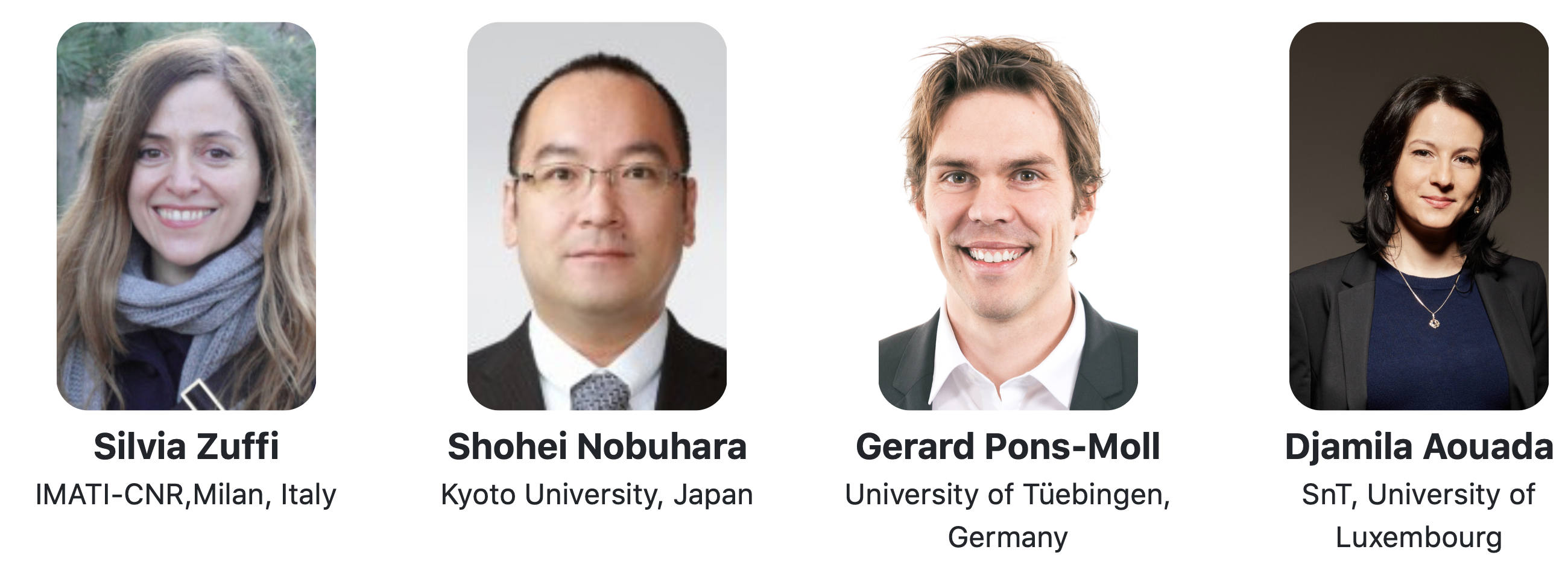
Time (CET) | Talks | Speakers |
Session 1 |
||
16:00 – 16:05 |
Opening Remarks |
Djamila Aouada, Kseniya Cherenkova |
16:05 – 17:00 |
Plenary Talk 1: Reconstructing deformable 3D surfaces from monocular video using geometry |
Adrien Bartoli |
17:00 – 17:55 |
Plenary Talk 2: Neural Implicits, Learning with Neural Distance Fields, NeRF and all that |
Gerard Pons-Moll |
17:55 – 18:00 |
Closing Session 1 |
Djamila Aouada |
Session 2 |
||
18:30 – 18:45 |
Challenge Presentation |
Djamila Aouada |
18:45 – 19:30 |
Presentations by Finalists |
Invited Challenge Participants |
19:30 – 19:35 |
Announcement of Results |
Kseniya Cherenkova |
19:35 – 20:00 |
Analysis of Challenge Results |
Anis Kacem |
20:00 – 20:25 |
Panel Discussion with invited panelists: Silvia Zuffi, Shohei Nobuhara, Gerard Pons-Moll |
Djamila Aouada (Moderator) |
20:25 – 20:30 |
Closing Remarks |
Djamila Aouada |
Plenary Talks
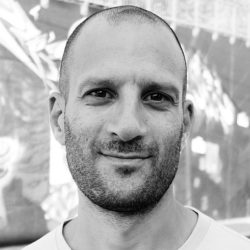
Prof. Adrien Bartoli
Plenary Talk 1: Reconstructing deformable 3D surfaces from monocular video using geometry
Abstract: It was established that 3D reconstruction from multiple images is solvable by Structure-from-Motion, where a moving camera films a rigid object. Structure-from-Motion has become a 3D reconstruction technology: one may for instance film a static object using a smartphone and convert the footage to a dense 3D point cloud automatically on a regular computer. The process may also compute the camera’s time-varying position, orientation and internal parameters, such as the focal length. However, how about deforming scenes and objects? Can one film a handheld, deforming piece of cloth and later recover 3D from the footage?
The question is not about using several synchronised cameras to observe a deforming object, which would boil down to the rigid case of Structure-from-Motion. It is about using images from a single camera and recovering dynamic, time-varying 3D structure from these images. The constraints involved in deformable 3D reconstruction are not only weaker than in rigid 3D reconstruction: they also make it a tremendously more involved problem. Research has nonetheless recently led to some important theoretical and practical results for the case of near-isometric structures. In short, deformable near-isometric structures can be uniquely 3D reconstructed from images in many practical cases. In this presentation, the methods of Shape-from-Template and Non-Rigid Structure-from-Motion will be discussed. Then, it will be shown how these methods facilitate augmented reality in laparoscopic surgery, providing the surgeon with live visual guidance in the operating theatre.
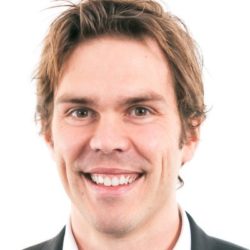
Prof. Gerard Pons-Moll
Plenary Talk 2: Neural Implicits, Learning with Neural Distance Fields, NeRF and all that
Abstract: The field of 3D shape representation learning and reconstruction has been revolutionised by combinations of neural networks with implicit and field representations. Most neural implicit models classify the occupancy or regress the signed distance to the surface for every point in space.
I will first describe IF-Nets which significantly improve the robustness and accuracy of previous neural implicits. Instead of classifying based on point-coordinates, IF-Nets extract multi-scale deep features from the input, allowing o reason about local and global shape properties. IF-Nets, however, like most other neural implicits, can only reconstruct closed surfaces — open manifolds, and shapes with inner structures (for example, garments, 3D scenes, cars with inner structures) can not be represented. To address this, I will describe Neural Distance Fields (NDF), which can output any kind of 3D shape, including open manifolds. I will show how NDFs can be used not only for 3D shape representation, but more generally for multi-modal regression. I will also draw connections to Energy Based models. If time allows, I will also describe our recent works in making NeRF dynamic, generalisable to novel scenes, and controllable.
Biography: Prof. Bartoli is a world expert in 3D data modelling and reconstruction. He is recognised and acknowledged by the computer vision community, serving regularly as Area Chair at the major venues. Indeed, Prof. Bartoli has a profound knowledge about non-rigid 3D data reconstruction. His perspective on the next steps in 3D data processing will surely open interesting discussions at SHARP 2021.
Biography: Prof. Pons-Moll is one of the leading researchers in 3D human body modelling. He has contributed to the most recent and successful models. He is also, along with his student, winner of the SHARP challenge in 2020. Prof. Pons-Moll is therefore familiar with the type of datasets presented at SHARP. His perspective and experience will undoubtedly make the audience of SHARP dive right into the real challenges.